L7 | CHATS
thought leadership
Fixing AI’s Blind Spot: The Role of Knowledge Graphs in Life Sciences
by Vasu Rangadass, Ph.D. | posted on March 25, 2025
The promise of AI in life sciences is undeniable: It can accelerate drug discovery, optimize manufacturing, and personalize patient care. Yet, despite all the advancements, I often hear the same frustration from executives, data scientists, and IT leaders: “We’ve invested in AI, but we’re not seeing the impact we expected.”
The problem isn’t AI itself—it’s data. Or rather, data silos.
AI models, particularly Large Language Models (LLMs), are only as good as the data they’re built on. And yet, life sciences data is fragmented, unstructured, and locked in silos across R&D, manufacturing, and clinical operations. AI that lacks context and governance doesn’t just produce inefficiencies—it creates risk.
I’ve seen firsthand how organizations struggle to extract real intelligence from their data. Without a foundation of structured, explainable, and trusted data, even the most advanced AI will fail to deliver reliable results. The key to unlocking AI’s potential lies in knowledge graphs and unified data platforms—technologies designed to bring order, context, and meaning to life sciences data.
AI Without Context is Just Guesswork
At its core, AI thrives on patterns—but it can only recognize valuable patterns if the underlying data is structured and meaningful. LLMs promise to unlock vast amounts of data but suffer from a critical flaw: lack of trust.
A recent ScienceDirect article, Knowledge Graphs as a Source of Trust for LLM-Powered Enterprise Question-Answering, highlights this challenge. LLMs, while powerful, have a hallucination problem—they can fabricate answers with no clear provenance. They lack the ability to verify, validate, or explain their responses.
This is a non-starter in life sciences—where regulatory compliance, patient safety, and scientific integrity are paramount. AI that cannot be trusted is worse than no AI at all.
The Role of Knowledge Graphs: Structure, Trust, and Explainability
Knowledge graphs address these challenges by imposing structure and context onto data, enabling AI to generate accurate, explainable, and governable insights. The solution isn’t just more data—it’s more smart data. Here’s why Knowledge Graphs matter:
- Accuracy: AI models trained on disconnected data are prone to errors. Knowledge graphs connect the dots, reducing hallucinations and increasing precision.
- Explainability: AI can’t be a black box in life sciences. Knowledge graphs provide a traceable lineage of information, showing how AI concluded.
- Governance: Compliance is non-negotiable. Knowledge graphs ensure AI-driven insights are sourced from approved, authoritative data.
BUT KNOWLEDGE GRAPHS ARE HARD TO CREATE FROM A LOT OF SILOS.
Unifying Data and Workflow Orchestrations: The Missing Piece
But Knowledge Graphs alone aren’t enough. To drive real impact, they must be operationalized within a unifying data and workflow orchestration platform. AI doesn’t just need structured data—it needs orchestrated and contextualized-at-the-time-of-execution data that seamlessly flows across scientific, operational, and business systems.
Generating Knowledge Graphs from siloed applications is expensive and difficult… and traditional methods don’t scale
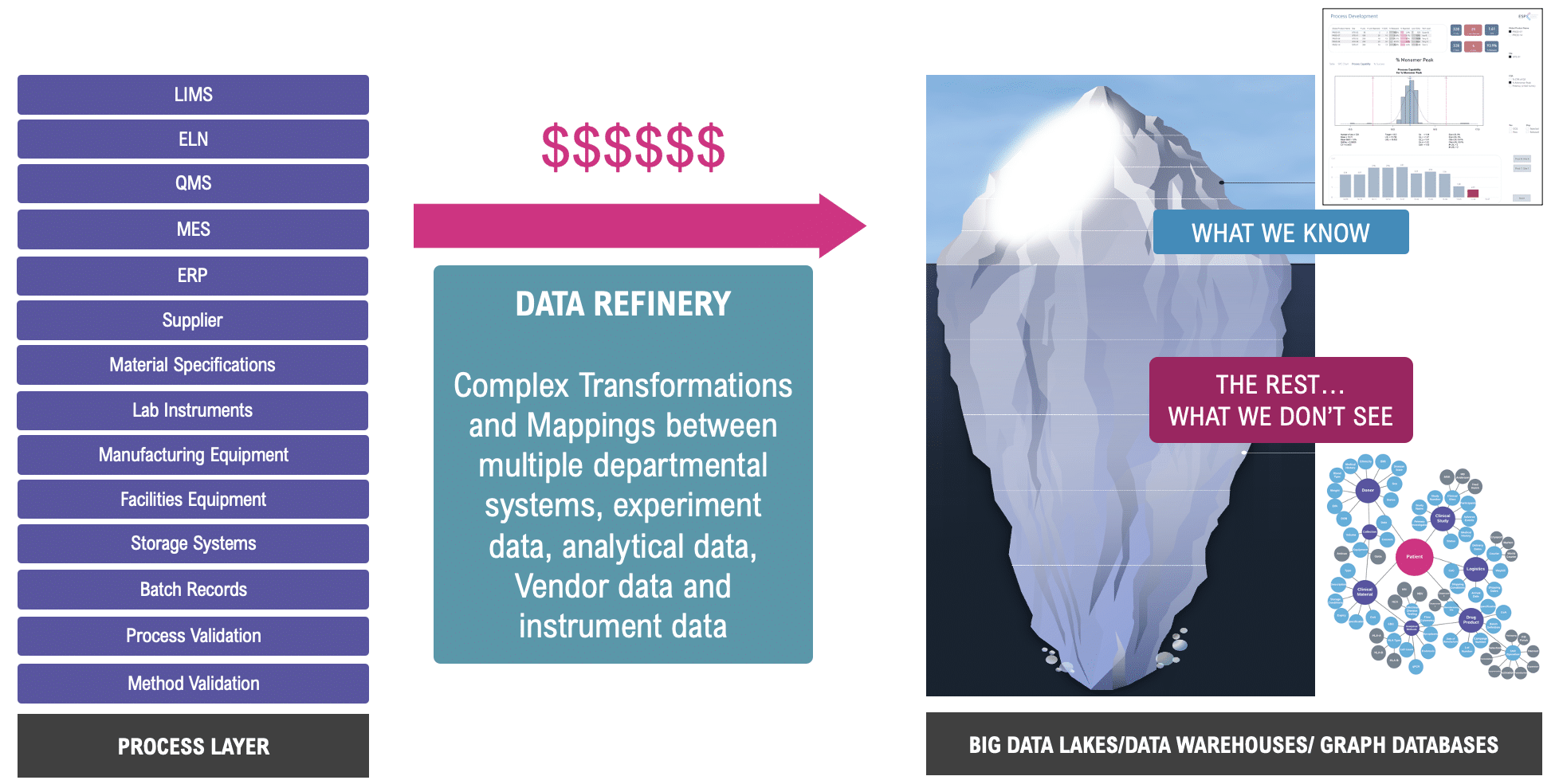
This is where proper orchestration platforms like L7|ESP® come into play. By unifying workflow automation, data contextualization, and Knowledge Graph generation (AI readiness), organizations can finally move from AI potential to AI execution.
Automatic data contextualization: the contextualized data layer enables the data model required for analytics and ML/AI tools
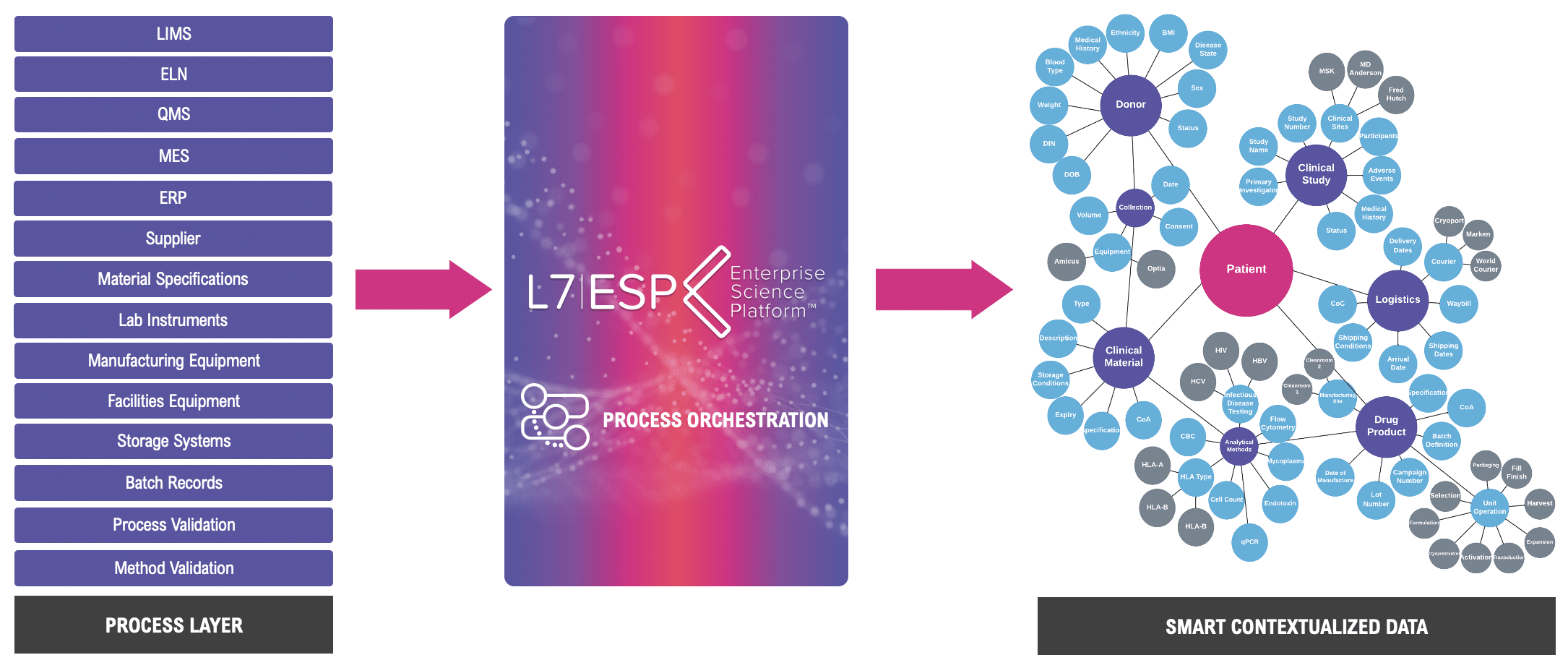
AI Without Data Contextualization is a Risky Bet
Organizations that fail to address data fragmentation and governance will remain stuck in expensive data harmonization and contextualization projects, fragmented insights, and compliance risks. The way forward is clear: AI must be built on a foundation of data orchestration engines that generate contextualized, structured, and explainable data.
Orchestration platforms can automate and contextualize data
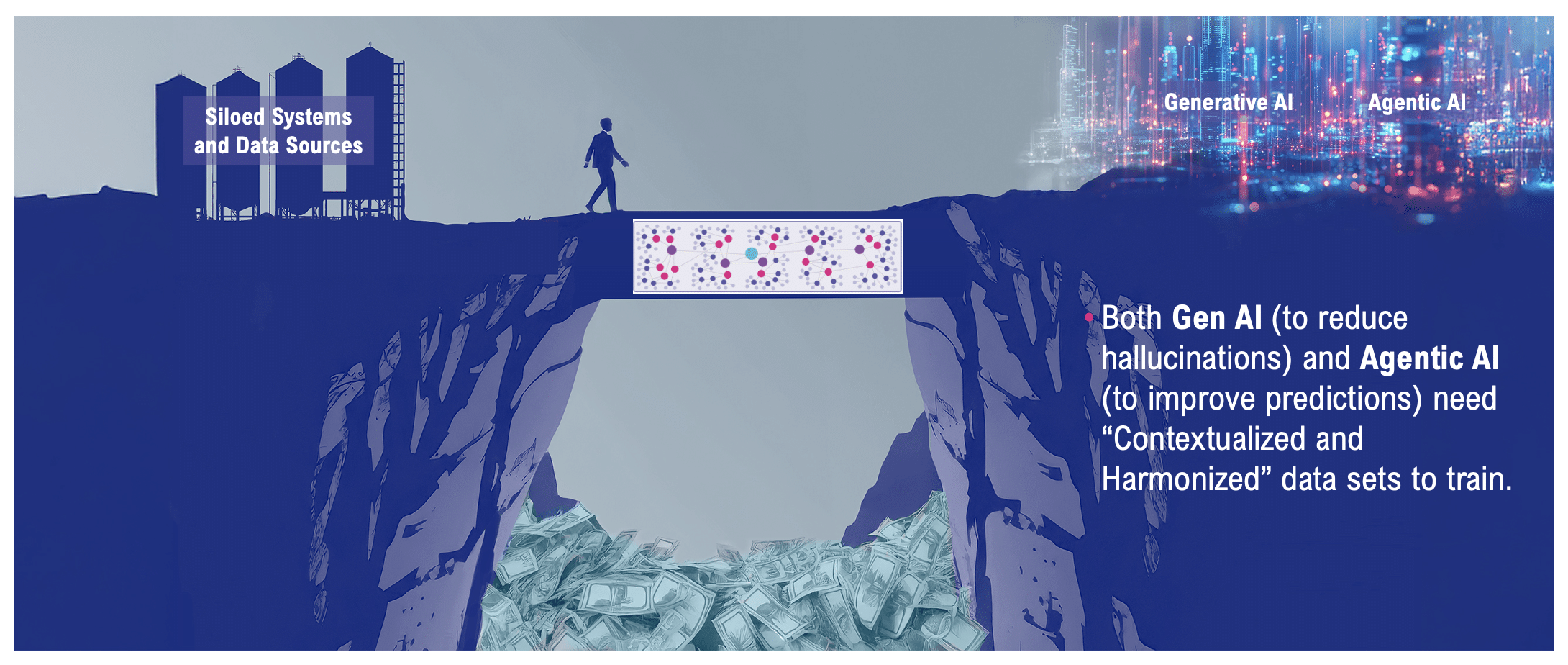
The technology exists. The strategy is clear. The only question is: How long can life sciences organizations afford to wait?
If you’re ready to dive deeper, check out our recent blog post “Bridging The AI Chasm in Life Sciences: Why Orchestration Platforms are the Key to Intelligence,” or explore our website at L7Informatics.com.