L7 | CHATS
‘everything data’ series
Building the Foundation for Artificial Intelligence Applications
by Brigitte Ganter, Ph.D. | posted on May 08, 2024
This post is the beginning of a series of blog entries that will focus on “everything data,” including how L7|ESP is well positioned to support complex laboratory and business process data applications, thus allowing organizations to make the best-informed business decisions in real time. We will cover new L7 Informatics data product launches and updates on critical partnerships L7 Informatics is engaging in (e.g., SciBite) for the benefit of our customers so they can overcome the challenges the life science industry is facing concerning the heterogeneity and complexity of language, terminology, and data sources.
Realizing The Potential of AI/ML with the Right Data
Access to artificial intelligence (AI) and machine learning (ML) technologies has broadened people’s visions and ambitions across many life sciences sectors. Certainly, AI increases the potential of data-driven insights delivery and, with that, carries the promise of process optimizations, especially for large, complex data. Furthermore, AI is lowering the barriers to entry for many, allowing users to extract insights from complex data without the need to write code or complex queries but rather take advantage of an AI interpretation layer (e.g., natural-language-based interpretation). A comparison can be drawn to the availability of CPU- and GPU-based computing, which resulted in a range of different computational developments that were not possible several years before. For example, before the cloud, large engineering teams were needed to support complex infrastructures, making it cost-prohibitive and inaccessible to all but a minority of large organizations. Cloud computing changed this, increasing compute accessibility and thus fueling new ambitions to utilize these capabilities with ever-evolving use cases.
Similarly, applications of AI/ML technologies are limitless, given the pre-requisite data corpus for model development and evaluation. However, the quality of this data has a huge impact on the accuracy and effectiveness of the model. AI/ML data modeling can be applied throughout the pharmaceutical value chain, including biomarker development, clinical trial optimization, drug repurposing, competitive intelligence research, post-market surveillance, and certainly lab and business process optimization.
The ability to apply any model to any type of data requires the data to be standardized, structured, organized, and readily accessible. Otherwise, the data is of no value to any data consumer.
Well-managed, Structured, and Standardized Data Is Foundational for Successful AI Applications
Siloed and fractured systems, complex data, and process architectures, coupled with the rapid expansion in unstructured data volume and complexity, have challenged the potential to implement AI and realize its true value. A unified data strategy covering alignment of terminologies, and flexible platform architectures is needed to extract laboratory and business process insights. These insights can then drive process optimization, shortening the overall product life cycle, and reducing costs.
To derive valuable insights from these data, the data must be contextualized, properly managed, and organized while also being easily findable, accessible, interoperable, and reusable, or in other words, following FAIR data guiding principles. Only then can the value be realized, for example, via developing predictive AI models that can be translated into new and improved lab and business processes.
FAIRification of data and processes within the L7|ESP Process Orchestration Platform via data integration and contextualization improves business intelligence, increases business velocity, and reduces costs.
A Data-Centric Approach is the Path Forward to Create Value for the Data Consumer
Data-centricity is also the solution to address the pharmaceutical industry’s ROI challenges.
Losing exclusivity of key assets, spiraling R&D costs, and a continuing need to demonstrate return on investment (ROI) have resulted in the pharmaceutical industry facing escalating pressures. To overcome these pressures, the industry actively adopted innovative high-throughput technologies, heavily invested in modernizing data infrastructure, and – more recently – in AI capabilities to derive insights from the rapidly growing volumes of increasingly complex data across the entire value chain. However, to derive high-value insights from an AI model requires high-quality and contextualized data as an input; otherwise, the saying “garbage in, garbage out” holds true. This point is further demonstrated in the article by Sequeda and team (2023), which showed that Large Language Models (LLMs) require contextualized data – represented over a knowledge graph – to provide higher accuracy for LLM-powered question-answering systems (see Figure 1).
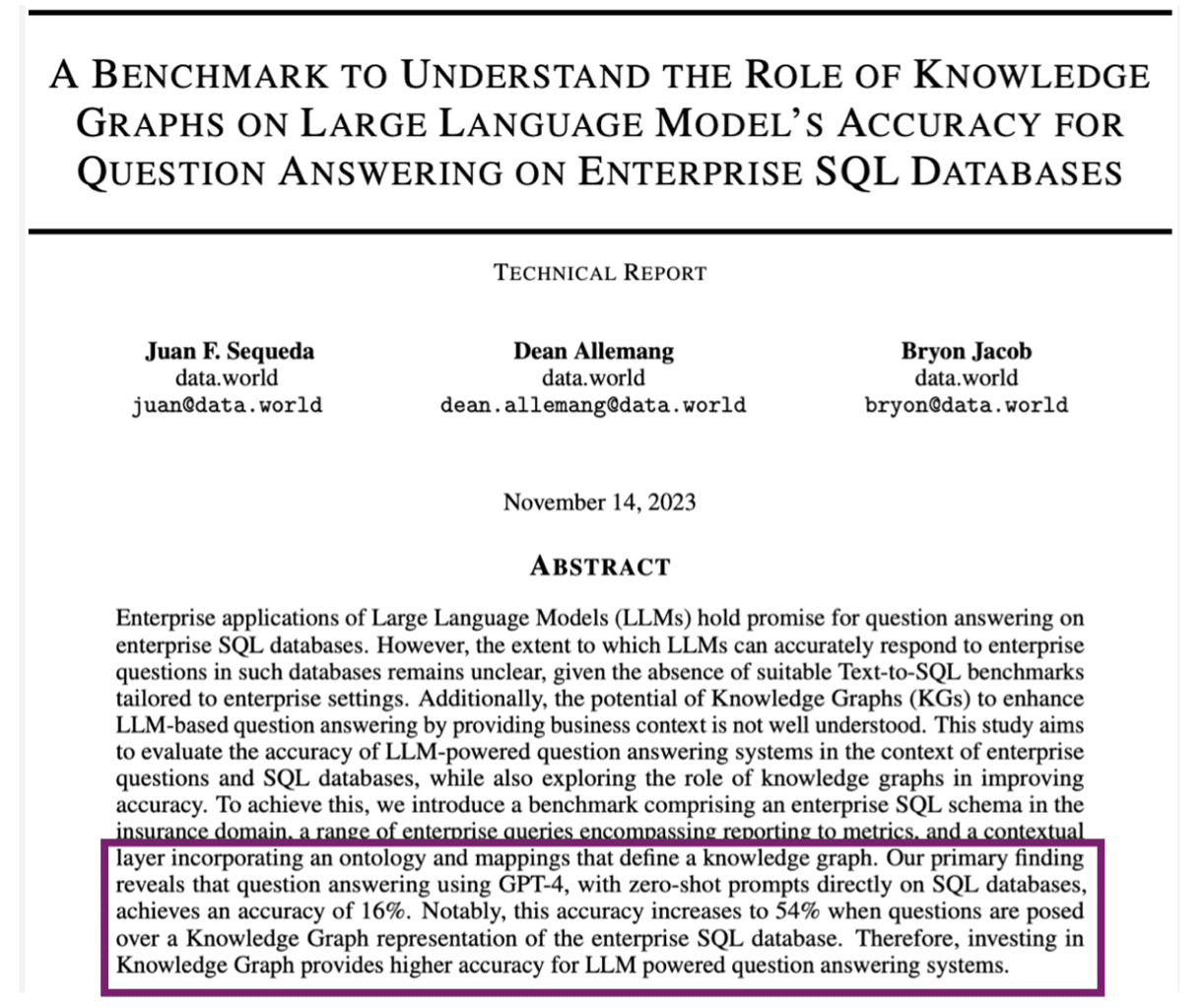
Figure 1: Investing in Knowledge Graph provides higher accuracy for LLM-powered question-answering systems.
Adding AI capabilities on top of uncontextualized, low-quality, and siloed data returns sub-optimal results, while data volumes and costs of storage are continuing to grow without an equivalent ROI expected from these new IT investments. Improved E2E (end-to-end) processes via optimized throughput, increased efficiency, with lower overall cost can only be achieved through a data-first approach. Well-structured and harmonized lab and process data and accompanying metadata needs must be captured at every stage and made accessible across the entire business. Only then can research and manufacturing bottlenecks be identified, and steps can be taken to optimize various process components or entire processes. Having access to these process insights allows pharma companies to achieve shortened product life cycles at an overall reduced cost.
To derive high-value insights from an AI model requires high-quality and contextualized data as an input.
L7|ESP Is an Automated and Unified Platform that Controls and Executes Regulated Laboratory and Business Workflows
The Unified Platform L7|ESP, with its Workflow Orchestration and Data Contextualization and its architecture for digitalization, not only integrates all common data information management systems, including LIMS, Notebooks, and MES together with other process-oriented applications, it also is open and flexible to integrate with existing management systems and even works with legacy systems providing a unified view of all laboratory and business processes across the value chain, increasing transparency of the product life cycle and its information flow, ensuring operational efficiency.
L7|ESP’s data and workflow orchestration not only automates, optimizes, and executes tasks, providing a holistic approach to scientific process and business management, it also harmonizes and structures data at the point of capture, which includes all master process data and contextualized meta data (see Figure 2 for the L7|ESP Knowledge Graph). As an additional benefit, it can also be extended with data trending and charting tools for real-time operational and scientific insight.
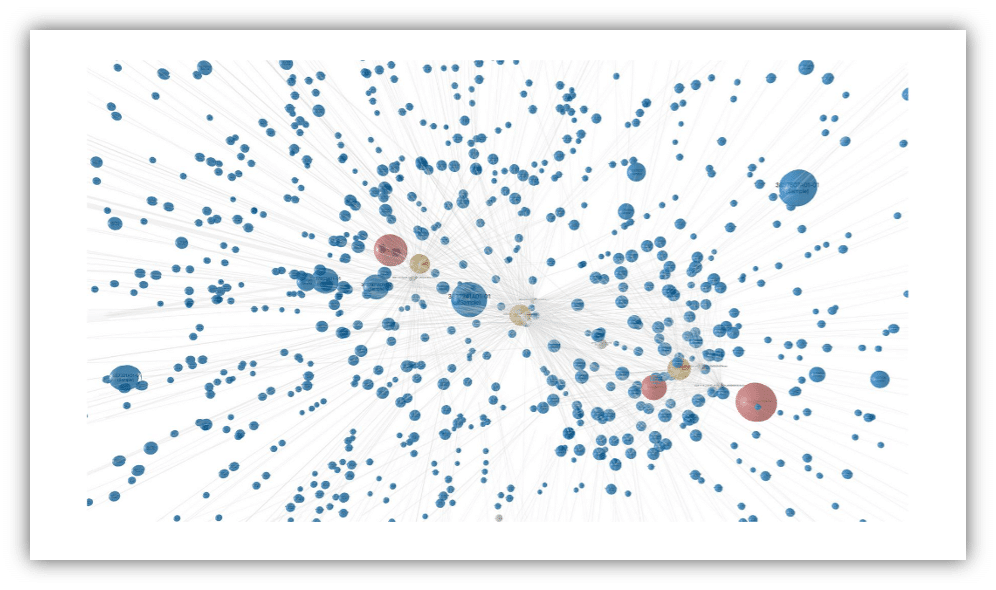
Figure2: L7|ESP Knowledge Graph.
A unified view of all laboratory and business processes across the value chain, as provided via L7|ESP, increases transparency of the product life cycle and its information flow, ensures operational efficiency, and reduces operational costs.
References
Sequeda et al., A Benchmark to Understand the Role of Knowledge Graphs on the Large Language Model’s Accuracy for Question Answering on Enterprise SQL Databases. (2023) arXiv:2311.07509 [cs.AI], Nov 13.